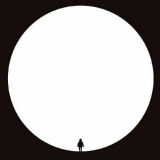
Radbrt
I am used to Snowflake. The last few years of my career has largely been focused on management and data engineering aspects of Snowflake. Now, I have to learn Databricks. Largely because Databricks doesn’t require a procurement process. It is procurement-driven architecture.
Shifting focus from a Database-first, python-as-an-afterthought platform to a Python-first, Database-as-an-afterthought platform is frustrating.
Snowflake has had it’s 15 minutes of infamy when it comes to security. I suspect it is a mere coincidence that the hacking crew was targeting snowflake instead of Databricks.
A few years ago, helped along by zero interest rates, the data space was buzzing. A lot of new companies, mostly SaaS, a lot of new features, frameworks and libraries. To borrow a phrase, it felt like running in front of a train.
Now, with higher interest rates and LLMs devouring most of the VC money, the data space is a lot quieter. There doesn’t seem to be a MAD data landscape this year, but if a new one comes we for the first time see fewer entrants - particularly if we omit the LLM-specific stuff.
Yes, that PoC: https://www.reddit.com/r/dataengineering/comments/1h2t8op/dbt_poc_in_our_company_ended_in_a_disaster/
Go read it if you haven’t. The comments too, although they are pretty much all saying the same thing.
In brief, the post describes the following:
Analytics team pushes through a dbt PoC Security team and everyone sits back and watches Analytics team deliver some dashboards in record time People discover numbers don’t match Analytics team discover the entire codebase is spaghetti People discover there is basically no access control on new tables And the comments basically go:
A few weeks back I came across https://european-alternatives.eu/, a site dedicated to highlighting European alternatives to digital services. The fact that such a site is needed is really sad, but it is an interesting read none the less.
And in the last few days, I have seen repeated calls for european digital sovreignty. The same call we have seen plenty of times before, but has received renewed attention after the US election.
It is about 10 months since I first wrote my post on dbt unit tests. That was before launch, before betas. dbt unit tests were released with dbt 1.8 in early may, and I have had the chance to do some real dbt development since then.
Why does it feel different now? 🔗It is not often I think of a minor release as transformative. But dbt 1.8 was. For the first time (in dbt, anyways), I could write tests as part of writing the logic.
The topic of test data comes up from time to time, and is plagued by the fact that test data can mean many different things. And that these things don’t have names.
A test data topology 🔗I have had the idea of a taxonomy of test data for a while. Like most taxonomies it won’t catch all nuances, or edge cases. And that is as much a feature as it is a bug.
The hype has subsided now, but you can still see it: The stack-fixation. Data teams comparing their data stacks, as if some magical combination of open-source and SaaS tools would solve all the problems. Fortunately few really believed a SaaS would save the world, but it could seem like it at times. Because tools are easy to talk about. The second easiest thing to talk about is how we shouldn’t talk about tools.
Today I changed visibility on my Metadog repository from private to public, and added an Apache 2 license. You can find it here: https://github.com/radbrt/metadog.
More comprehensive introductions are hopefully to come, but I wanted to introduce it and explain what and why.
Why Metadog 🔗I made Metadog as part of my job as a data engineer, where I needed to keep track of data on a number of different upstream systems (databases, SFTP servers, blob storage…) as well as in our own databases.
One of the many corners of the (post-)modern data stack I have kept an eye on is observability. I recently revisited it, and while little has changed, much has changed.
At its core, observability is about process monitoring. Finding changes, because changes might be errors. Perhaps interestingly, status quo is rarely suspected to be an error.
Mostly, observability is about finding changes in data. Changes in row counts. Changes in distinct values.
I started writing this post a while back, but now that it has stayed half-done for several months I’m posting what I have.
I wrote about CLIP models a while back, but from a high-level “what are they and what can they be used for” perspective. Now I have had the chance to work more with clip models directly in python, and they are still impressive.
You can use clip models with the transformers library from huggingface, there are special CLIPModel and CLIPProcessor classes:
← previous | This site is part of the Data People Writing Stuff webring random | index | what is this? |
next → |